
XAI for Predictive Maintenance
Tutorial at ECAI 2023
26th European Conference on
Artificial Intelligence ECAI 2023
​
Kraków, Poland
​
Sat, Sep 30, 2023 – Thu, Oct 5, 2023
Presenters:
-
Joao Gama, University of Porto
-
Sławomir Nowaczyk, Halmstad University
-
Sepideh Pashami, Halmstad University
-
Rita P. Ribeiro, University of Porto
-
Bruno Veloso, University of Porto
-
Szymon Bobek, Jagiellonian University
​
Publicity chair:
-
Carlos Ferreira, LIAAD INESC TEC
Abstract
Explainable Predictive Maintenance (PM) focuses on creating methods that can explain the operation of AI systems within the PM domain. What makes creating the maintenance plans challenging is incorporating the AI output into the human decision-making process and integrating it with human expertise. To make AI helpful and trustworthy means putting fault predictions in a relevant context and making them understandable for the humans involved. It requires explanations that are adapted to the roles and needs of different actors – for example, to lower-level engineers through connection to the blueprints of the technical installation; while using other means for the managers who evaluate the costs of system downtime; or company lawyers assessing the possible liability in the case of safety-threatening failure.
​
Today, in many industries, various AI systems, often black-box ones, predict failures based on analyzing sensor data. They discover symptoms of imminent issues by capturing anomalies and deviations from typical behavior, often with impressive accuracy. However, PM is part of a broader context. The goal is to identify the most probable causes and act to solve the problem before it escalates. In complex systems, knowing something is wrong (i.e., detecting an anomaly) is not enough; the key is understanding its causes and potential consequences, estimating the remaining useful life, and providing alternatives to mitigate those consequences. However, this often requires complex interactions among several actors in the industrial and decision-making processes. Doing it fully automatically is unrealistic, despite recent impressive progress in AI. This tutorial aims to present current trends and promising research directions within Machine Learning for Predictive Maintenance. In addition, we will present some of the state-of-the-art methodologies in Explainable AI (XAI) relevant to predictive maintenance problems.
Further, we will provide hands-on examples of applying XAI in benchmark datasets. This year the focus will be on explainable predictive maintenance (XPM). We will also discuss future challenges and open issues on this topic. The tutorial will discuss four case studies related to Predictive Maintenance challenges in the Metro operation, Steel factories, and Wind farms.
Bios
Joao Gama
is a Full Professor at the School of Economics, University of Porto, Portugal. He received his PhD in Computer Science from the University of Porto in 2000. He is a EurIA Fellow, IEEE Fellow, Fellow of the Asia-Pacific AI Association, and a member of the board of directors of the LIAAD, a group belonging to INESC TEC. He is ACM Distinguish Speaker. His h-index at Google Scholar is 68. He is an Editor of several top-level Machine Learning and Data Mining journals. He served as Program Chair of ECMLPKDD 2005, DS09, ADMA09, EPIA 2017, DSAA 2017, Conference Chair of IDA 2011, ECMLPKDD 2015, DSAA’2021, and a series of Workshops on KDDS and Knowledge Discovery from Sensor Data with ACM SIGKDD. His main research interests are knowledge discovery from data streams, evolving network data, probabilistic reasoning, and causality. He published more than 300 reviewed papers in journals and major conferences. He has an extensive list of publications in data stream learning.
Sławomir Nowaczyk
Center for Applied Intelligent Systems Research, Halmstad University, Sweden. SÅ‚awomir is a full professor in Machine Learning and a Research Leader for Embedded and Intelligent Systems environment at the School of Information Technology. He has a PhD degree from Lund University of Technology, Sweden. During the last decade, his research focused on knowledge representation, data mining, and self-organizing systems, especially in large and distributed data streams, including unsupervised modeling. Together with over ten different industrial partners, he was involved, often in leading roles, in close to twenty research projects related to predictive maintenance, diagnostics, and self-monitoring. He is a board member of the Swedish AI Society, served as a reviewer for 15+ journals and 50+ conferences, and has extensive experience in organizing events, for example, the Scandinavian Conference on Artificial Intelligence 2015 in Halmstad or Transparent, Explainable and Affective AI in Medical Systems workshop at AIME 2019. He has co-organized the previous editions of this tutorial, at ECML 2019 and 2020, as well as the relevant special session and summer school at DSAA’2021.
Sepideh Pashami
is a senior researcher at RISE and a lecturer at the Center for Applied Intelligent Systems Research, Halmstad University. She received her PhD degree from AASS Research Centre, ¨ Orebro University, Sweden, in 2016. Sepideh enrolled as the Technology Area Leader for Aware Intelligent Systems part of the Intelligent Systems and Digital Design department in 2020. Her research interests include predictive maintenance, interactive machine learning, causal inference, and representation learning. She has been involved as a researcher and research leader in several projects (e.g. EVE, In4Uptime, ARISE, and HEALTH) together with Volvo Group AB, applying machine learning techniques for predictive maintenance of heavy-duty vehicles. She served on the organizing committee of the workshop ECML/PKDD 2019-2020, IDM-WSDM 2019 workshop, industry days at IJCAI-ECAI 2018, RoboCup in IranOpen2008, and IranOpen2009. She serves as a reviewer for IEEE Transactions on Intelligent Transportation Systems, Sensors, and the journal Science of Computer Programming.
Grzegorz J. Nalepa
is an engineer with degrees in computer science - artificial intelligence, and philosophy. He has been working in the area of intelligent systems and knowledge engineering for over 15 years. He formulated the eXtended Tabular Trees rule representation method, as well as the Semantic Knowledge Engineering approach. He authored the book ”Modeling with Rules using Semantic Knowledge Engineering” (Springer 2018). He co-edited the book ”Synergies Between Knowledge Engineering and Software Engineering” (Springer 2018). He co-authored over 150 research papers in international conferences and journals. He coordinates GEIST - Group for Engineering of Intelligent Systems and Technologies (http://GEIST.re) and cooperates with Jagiellonian University and AGH University in Krakow, Poland. He is a member of the Polish Artificial Intelligence Society (PSSI), IEEE, Italian Artificial Intelligence Society (AI*IA), and KES. For almost 10 years he has been co-chairing the Knowledge and Software Engineering Workshop (KESE) at KI, the German AI conference, Spanish CAEPIA, as well ECAI. He was the guest editor in several JCR journals, including the International Journal of Applied Mathematics & Computer Science (AMCS), the International Journal of Human-Computer Studies (IJHCS), and Future Generation Computer Systems (FGCS). In 2020 he founded Jagiellonian Human-Centered AI Laboratory (JAHCAI). His recent interests include context-aware systems, affective computing, and explainable AI. Recently he also organized two editions of the AfCAI workshop in Murcia (2016), Valencia (2018), Cartagena (2019), the XAILA workshop at the Jurix conference in 2018 and 2019, and the MIEL workshop on IDEAL 2018 and BPM2019.
Rita P. Ribeiro
is an Assistant Professor at the Department of Computer Science of the Faculty of Sciences of the University of Porto (FCUP) and a Researcher at the Artificial Intelligence and Decision Support Lab (LIAAD) of the Institute for Systems and Computer Engineering, Technology and Science (INESCTEC). She holds a PhD in Computer Science from the University of Porto. Her main research topics are predictive learning from imbalanced domains, evaluation issues on learning tasks, and topics related to social good in AI. She has been involved in several research projects concerning environmental problems, fraud detection, and predictive maintenance applications. She is a member of the program committee of several conferences, an action editor of Machine Learning Journal, and a reviewer of several other journals. She has been involved in the organization of several scientific events: Workshops and Tutorials held in conjunction with ECML PKDD (STREAMEVOLV2016, IOTStreaming 2018, LIDTA 2020, SoGood 2020-2022, IOTstream 2022), Doctoral Consortium Chair IDA 2021, Tutorial Chair at DSAA 2021, Summer Schools (EAIA 2018 - ”Advanced School on Data Science for Big Data, Summer School on Data-Driven Predictive Maintenance for Industry 4.0 - colocated with DSAA 2021), Program Chair of DS 2023.
Bruno Veloso
is a senior researcher at LIAAD and an assistant professor at the Faculty of Economics, University of Porto. He received his PhD from the University of Vigo, Spain 2017. His research interests include data mining, machine learning, and predictive maintenance. He has worked on a project together with Metro of Porto. He is a member of the program committee of several conferences and a reviewer of several other journals.
Szymon Bobek
is an Assistant Professor and a member of the GEIST research team at Jagiellonian University. He participated in several international research projects where he was involved in tasks concerning knowledge engineering and rule-based inference. His current research interests are machine learning, ambient intelligence, context awareness, and knowledge engineering.
Carlos Ferreira
(publicity chair)
My main research interests are related to Machine Learning, Pattern Mining, Deep Learning and Causality. My focus is on predictive analytics and outlier detection tasks, in problems that may involve temporal and non-stationary data in Agriculture and Health Care fields.
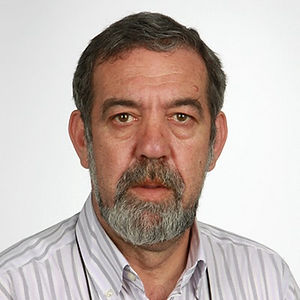
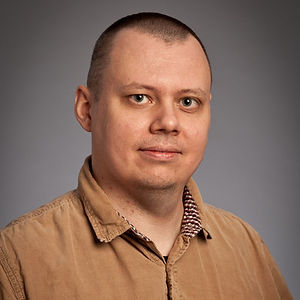
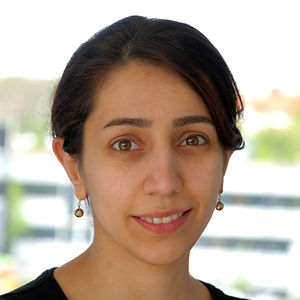
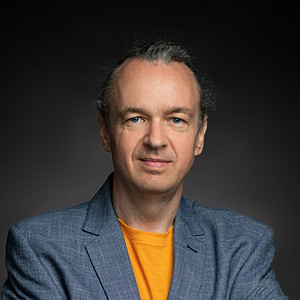
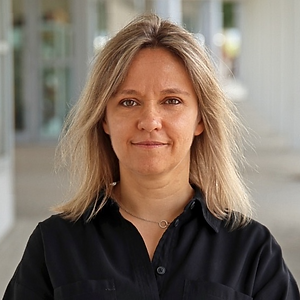

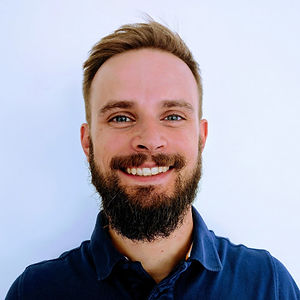
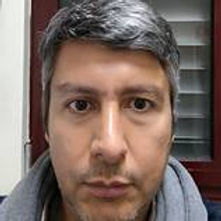
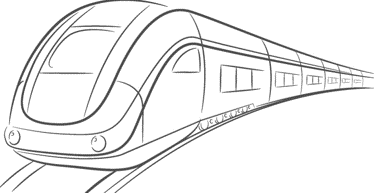
Competition
The compressed air system is a crucial component of the train and delivers essential pressurized air. The competition task is to predict a failure or detect an anomaly two hours in advance (minimum time required by the company to schedule the replacement of the faulty train).
The dataset consists of more than 15 million examples collected from February to August 2020, with 15 features from 7 analog and 8 digital sensors.