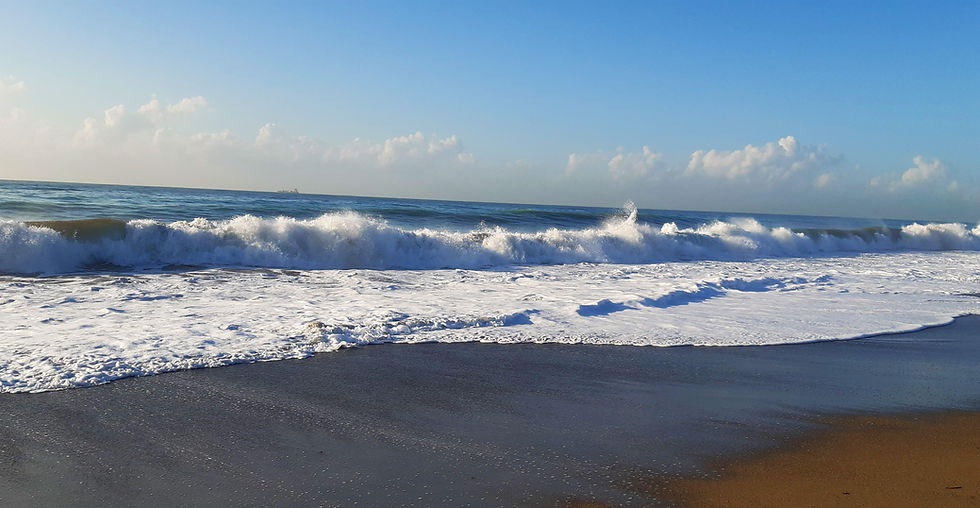
XAI for Predictive Maintenance
Lecture-style tutorial at KDD 2023
2023 ACM SIGKDD International Conference on Knowledge Discovery and Data Mining
Long Beach, CA, USA
Sun, Aug 6, 2023 – Thu, Aug 10, 2023
-
Joao Gama, University of Porto
-
Sławomir Nowaczyk, Halmstad University
-
Sepideh Pashami, Halmstad University
-
Szymon Bobek, Jagiellonian University
-
Rita P. Ribeiro, University of Porto
Abstract
Explainable Predictive Maintenance (PM) focuses on creating methods that can explain the operation of AI systems within the PM domain. What makes creating the maintenance plans challenging is incorporating the AI output into the human decision-making process and integrating it with human expertise. To make AI helpful and trustworthy means putting fault predictions in a relevant context and making them understandable for the humans involved. It requires explanations that are adapted to the roles and needs of different actors – for example, to lower-level engineers through connection to the blueprints of the technical installation; while using other means for the managers who evaluate the costs of system downtime; or company lawyers assessing the possible liability in the case of safety-threatening failure.
​
Today, in many industries, various AI systems, often black-box ones, predict failures based on analyzing sensor data. They discover symptoms of imminent issues by capturing anomalies and deviations from typical behavior, often with impressive accuracy. However, PM is part of a broader context. The goal is to identify the most probable causes and act to solve the problem before it escalates. In complex systems, knowing something is wrong (i.e., detecting an anomaly) is not enough; the key is understanding its causes and potential consequences, estimating the remaining useful life, and providing alternatives to mitigate those consequences. However, this often requires complex interactions among several actors in the industrial and decision-making processes. Doing it fully automatically is unrealistic, despite recent impressive progress in AI. This tutorial aims to present current trends and promising research directions within Machine Learning for Predictive Maintenance. In addition, we will present some of the state-of-the-art methodologies in Explainable AI (XAI) relevant to predictive maintenance problems.
Further, we will provide hands-on examples of applying XAI in benchmark datasets. This year the focus will be on explainable predictive maintenance (XPM). We will also discuss future challenges and open issues on this topic. The tutorial will discuss four case studies related to Predictive Maintenance challenges in the Metro operation, Steel factories, and Wind farms.
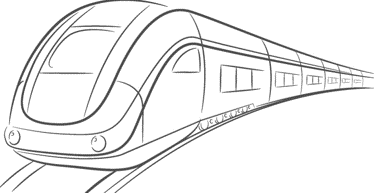
Competition
The compressed air system is a crucial component of the train and delivers essential pressurized air. The competition task is to predict a failure or detect an anomaly two hours in advance (minimum time required by the company to schedule the replacement of the faulty train).
The dataset consists of more than 15 million examples collected from February to August 2020, with 15 features from 7 analog and 8 digital sensors.